PhD Candidate in Information Technology – Systems and Control at Politecnico di Milano
ODYS Srl
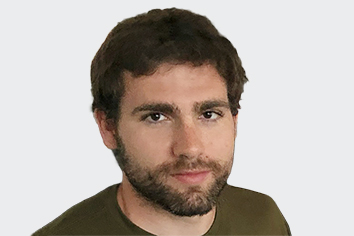
Kristoffer Løwenstein graduated from Aarhus University with a degree in mechanical engineering in January 2021. He worked with soft robotics in his thesis. His major research interests lie within dynamics, robotics, controls, artificial Intelligence, and the interplay between these fields. Kristoffer has carried out exchange semesters at Royal Melbourne Institute of Technology and Politecnico di Milano. Besides his studies, he played basketball at Bakken Bears winning multiple national championships in Denmark.
Project description
Next-generation embedded control systems in vehicles will have to cope with tight constraints on computation and storage resources, while at the same time satisfying increasingly challenging requirements on performance quality, energy consumption, safety, emissions, etc. In this context, model-based control techniques such as MPC are envisioned to play a key role, by providing a systematic framework to minimize a desired objective function under constraints. Real application of online MPC to high-volume automotive production programs has only very recently started to blossom and will likely continue to spread in the next years. For automotive suppliers of services and software like ODYS, it is essential to devote relevant resources to ease the design of MPC controllers and improve their performance, by reducing calibration time before production and adapting calibration parameters at run-time. To achieve these goals, the following three elements are equally important and demanding in terms of resources: (1) on-line adaptation of prediction models to cope with part-to-part variations, system aging and wear and tear of components, and to diagnose malfunctions of sensors and actuators, (2) use of reinforcement learning and policy search methods for self-tuning of MPC controllers, (3) a solution algorithm for the resulting optimization problem. Being able to perform the above three operations efficiently, and in a way that they are strictly intertwined, is key to enable the next-generation MPC modules in industrial production programs in the automotive industry. He will address several aspects of the above mentioned challenges, pursuing both theoretical research and application of the research results to embedded industrial use cases.
The expected outcomes of the research project are: (1) novel techniques for adaptive nonlinear MPC based on online machine learning, (2) control-oriented sparse optimization that allows one to trade off accuracy of the solution with speed of computation and memory occupancy, (3) tailored software implementation for highly resource-constrained embedded devices.