Research Engineer Associate, PhD candidate in Engineering Sciences at KU Leuven
Siemens Digital Industries Software
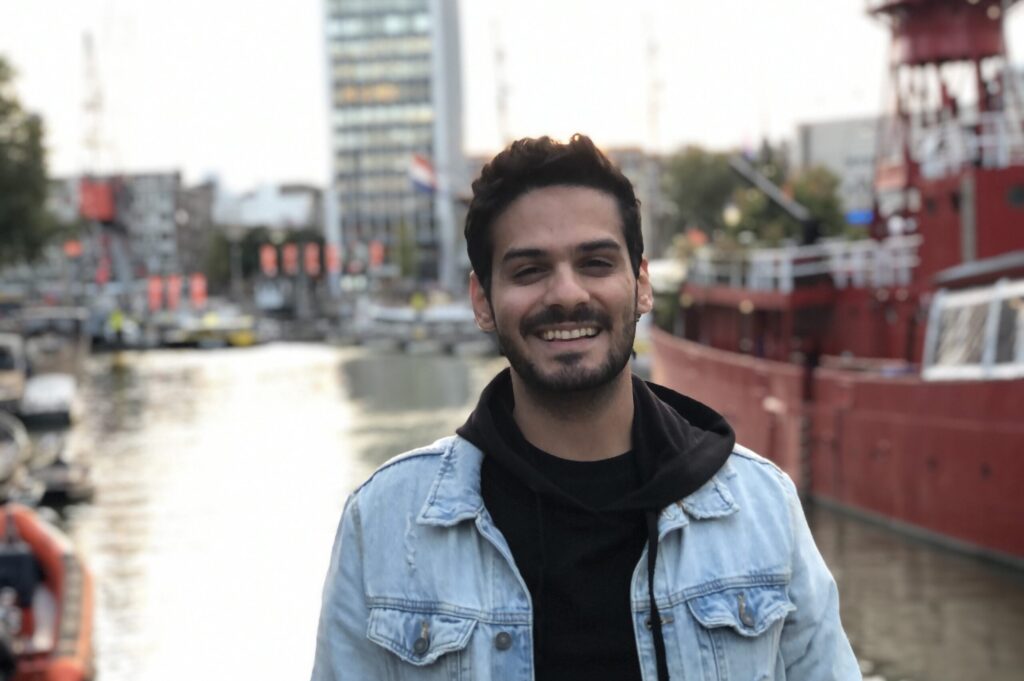
Jean Pierre Allamaa is a Lebanese student who graduated with a Masters in Mechanical Engineering with a specialization in mechatronics and control from Ecole Polytechnique Federale de Lausanne, Switzerland (EPFL) in 2020. His master thesis was about development and deployment of real-time optimal control for autonomous driving on road vehicles. Passionate about racing sports, he worked from September 2020 to June 2021 on NMPC and embedded optimization for competitive autonomous racing.
Project description
Jean Pierre will work towards a novel methodogical and embedded implementation development, which combines both optimal control and learning for safety objectives to increase the AD acceptability by developing advanced optimization and machine learning methods that are able to address challenging safety and comfort problems in autonomous driving. Safety is considered as the main driver for AD and ADAS development. While several existing assistance functionalities have proven their capabilities in simple use cases (e.g. adaptive cruise control), the automotive industry is continuously dealing with more safety-critical scenarios such as emergency lane change and intersection crossing. Today, most common control designs in the automotive industry rely on model-based non-optimal methods. They sometimes struggle to deal with safety-critical scenarios. Those who choose to develop with optimal control, face the challenge of real-time performance. Comfort, or the occupant’s perception of the vehicle performance in ADAS scenarios, is another challenge for automotive OEMs. Customers will only accept the ADAS functions if they experience comfortable feelings, and do not urge to take over vehicle control. Though significant knowledge is available on the performance perception for human drivers, these previous studies are no longer applicable for ADAS scenarios where the focus is on the occupant. Recently, machine learning techniques have shown advantages in several AD control applications. Methods such as imitation learning can introduce human likeness in the world of controls. However, they show limitations due to their lack of fundamental and rigorous results on explainability, safety and stability. Therefore, this PhD will focus on combining both optimal control and machine learning for embedded autonomous driving applications.